Transforming Business with Machine Learning Data Labeling
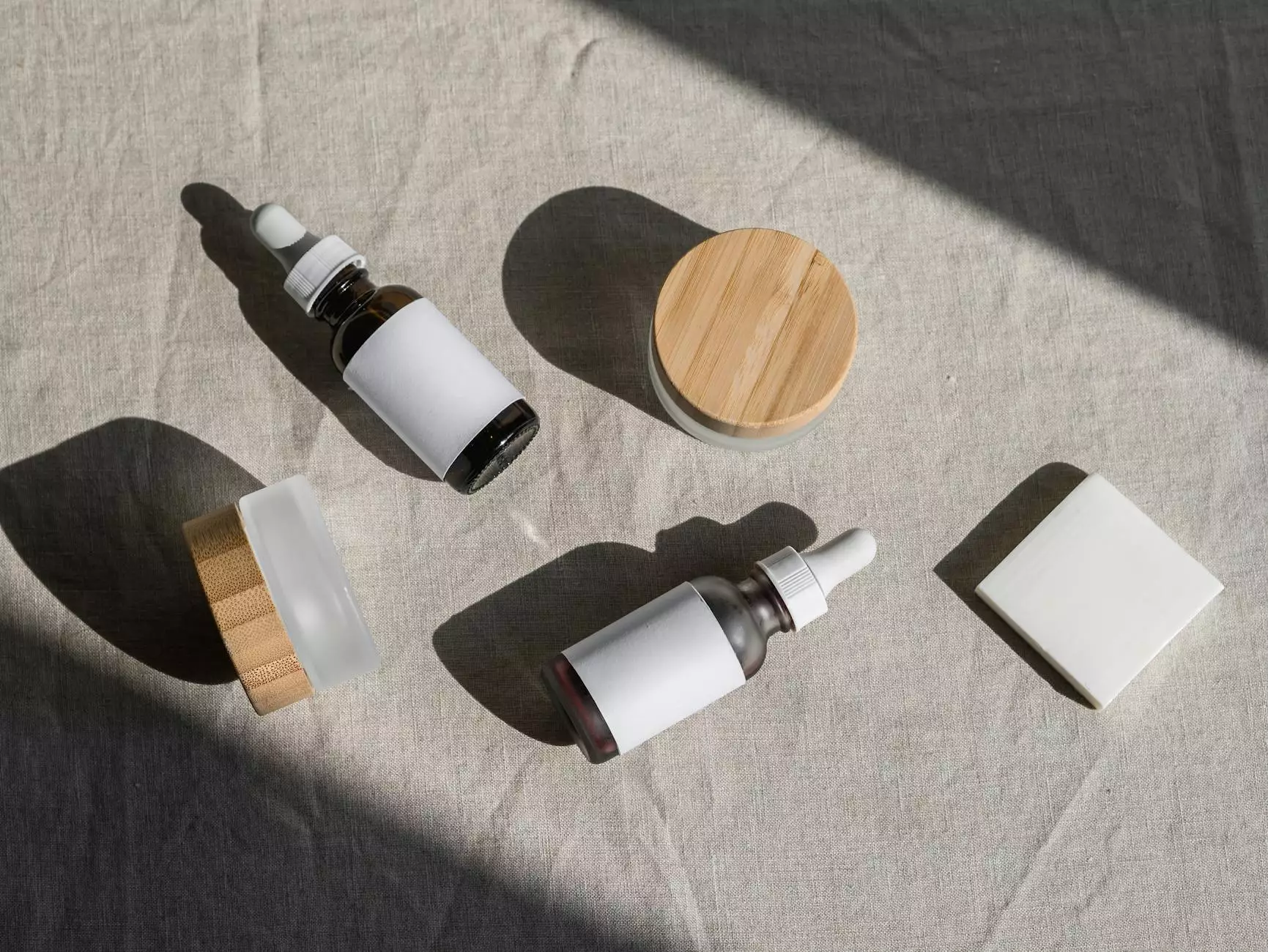
In the rapidly evolving landscape of technology, machine learning data labeling has emerged as a cornerstone of artificial intelligence applications. Businesses across various sectors are leveraging this powerful technique to refine their processes and enhance customer experiences. This article explores the significance of machine learning data labeling, its applications, the tools available, and how it's revolutionizing industries.
Understanding Machine Learning Data Labeling
Machine learning data labeling, at its core, is the process of annotating data to provide meaningful information that machine learning models can learn from. In simple terms, data labeling involves categorizing and tagging data so that algorithms can make sense of it, enabling them to predict outcomes or categorize new data points effectively.
The Importance of Data Annotation
Data annotation is vital for building high-quality datasets that machine learning models depend on. The accuracy of a model is significantly affected by the quality of data it has been trained on. Here's why data annotation matters:
- Model Performance: Properly labeled data leads to better-performing models, which translates into effective and reliable predictions.
- Reduced Bias: By ensuring diverse and accurately labeled datasets, businesses can mitigate bias in machine learning algorithms.
- Enhanced User Experience: Improved models contribute to products that better meet user needs, fostering customer satisfaction.
Applications of Machine Learning Data Labeling
The applications of machine learning data labeling span across multiple industries. Below are a few notable examples:
1. Healthcare
In healthcare, machine learning models rely on accurately labeled data to assist in diagnosis, treatment recommendations, and predictive analytics. Labeled medical images, for instance, can help models differentiate between healthy and abnormal tissues.
2. Autonomous Vehicles
For autonomous vehicles, data labeling is critical for training models to recognize objects on the road. Proper annotation of images and videos can help ensure that vehicles can safely navigate urban environments.
3. Retail and E-commerce
In retail, machine learning data labeling can enhance product recommendations and customer service through sentiment analysis and natural language processing. Annotated customer reviews can provide insights into product performance and customer satisfaction.
4. Financial Services
Fraud detection systems rely heavily on labeled datasets to identify unusual patterns in transactions. Effective data labeling helps in training models that can detect fraudulent activities in real-time, protecting businesses and consumers alike.
Choosing the Right Data Annotation Tool
With the growing demand for machine learning data labeling, numerous data annotation tools have entered the market. When selecting a data annotation tool, consider the following:
- User Friendliness: Choose a tool that is easy to navigate and supports your team's workflow.
- Flexibility: The tool should allow for a variety of data types including images, text, audio, and video.
- Scalability: As your dataset grows, the tool should be able to handle increased volumes without compromising performance.
- Integration Capabilities: The best tools can integrate with existing systems or frameworks, enhancing efficiency.
- Quality Assurance Features: Look for tools that offer built-in quality assurance mechanisms to ensure high labeling accuracy.
Key Features of Data Annotation Platforms
Data annotation platforms come with specific functionalities that cater to the unique needs of businesses. Here are some common features:
- Collaboration Tools: Effective platforms provide features that enable team collaboration, facilitating smooth communication and feedback processes.
- Custom Workflows: Teams can often create custom workflows tailored to their unique annotation needs, enhancing productivity.
- Automation: Advanced platforms offer automation options that can significantly speed up the labeling process, leveraging AI to assist in the task.
- Data Security: Robust security features ensure that sensitive data is protected throughout the annotation process.
Best Practices for Machine Learning Data Labeling
To achieve optimal results from machine learning data labeling, consider the following best practices:
1. Define Clear Guidelines
Establish clear and concise guidelines for labeling your data. This ensures consistency across annotated datasets and helps labelers understand expectations.
2. Use Multiple Annotators
Utilizing multiple annotators per data point can enhance accuracy, as differing perspectives can catch errors and biases that a single annotator might miss.
3. Continuous Training
Providing ongoing training and feedback to annotators can improve their skills over time, leading to higher quality annotations.
4. Regular Quality Checks
Implement routine quality assurance checks to ensure that the labeled data meets the required standards. This can involve reviewing a subset of annotated data to assess accuracy and consistency.
The Future of Machine Learning Data Labeling
As artificial intelligence continues to mature, the landscape of machine learning data labeling will evolve as well. Here are a few trends to watch for:
- Increased Use of Automation: More companies will rely on automated labeling tools to handle repetitive tasks, allowing human annotators to focus on more complex data.
- Improved Collaboration: As remote work becomes more prevalent, platforms that enhance collaboration among teams will gain traction.
- Rich Data Sources: Companies will increasingly explore diverse data sources to train their models, leading to more robust and versatile AI systems.
Conclusion
In conclusion, machine learning data labeling is not merely a technical requirement; it’s a strategic advantage that can propel businesses forward. By investing in high-quality data annotation tools and adhering to best practices, companies can streamline processes, improve product offerings, and ultimately enhance customer satisfaction. As technology continues to advance, those who embrace the power of machine learning and data labeling will undoubtedly stay ahead of the competition.
For businesses seeking to refine their machine learning capabilities, exploring platforms like KeyLabs.ai is a prudent step towards harnessing the full potential of data annotation.